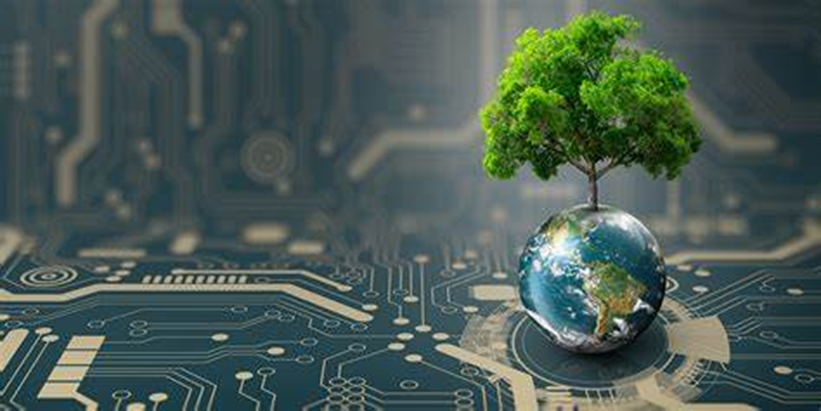
The Role of Climate Models and AI at COP29
At the COP29 climate conference, held this year in Baku, Azerbaijan, delegates and world leaders are engaged in high-stakes discussions centered around the financial and logistical challenges of climate mitigation. Central to the debates are climate models, highly complex computer simulations that aim to predict the trajectory of climate change and estimate the costs of necessary adaptations. Although these models have improved significantly since the United Nations first started organizing these summits, they are far from perfect, and their limitations present challenges in deciding how much financial aid to allocate to countries most vulnerable to climate impacts. These nations, often lacking resources to combat the effects of climate change, await decisions that hinge on the accuracy of models attempting to predict variables such as sea-level rise and seasonal shifts.
The primary models utilized in global climate discussions belong to the Coupled Model Intercomparison Project (CMIP), which since 1995 has coordinated over 100 models created by teams worldwide. CMIP’s goal is to capture climate impacts by dividing the world and atmosphere into grid-like cells, each represented by equations that simulate how these areas might respond to changing climate conditions. However, early versions of these models were limited by computational power, often rendering cell sizes in hundreds of kilometers—accurate enough to predict changes on a continental scale but unable to focus on specific nations or regions. As computational power has grown exponentially, modern models can now render cells of approximately 50 kilometers, providing more precise forecasts.
Despite these advances, some key climate processes remain hard to capture. For instance, clouds are challenging to model accurately due to their small scale and the profound influence even slight changes in cloud behavior can have on global temperature projections. Improving data inputs could help overcome some of these issues, but more immediate progress may come from harnessing artificial intelligence (AI) to enhance model performance. Companies like Google have taken the lead in integrating AI into climate prediction, introducing NeuralGCM, an advanced AI model trained on four decades of weather data. This model not only matches the performance of traditional forecasting tools but could also potentially surpass them in speed and efficiency.
AI's Emerging Role in Climate Modeling
Google claims that its AI model could soon make longer-term projections with greater certainty in areas like monsoons and tropical cyclones, processes notoriously difficult for traditional models to predict. NeuralGCM’s approach differs from traditional models by side stepping some physical complexities, instead relying on machine learning’s ability to detect patterns in vast datasets. However, some experts remain skeptical. Gavin Schmidt of NASA’s Goddard Institute for Space Studies warns that climate modeling faces unique challenges compared to short-term weather forecasting, given the need to simulate unprecedented climate conditions over extended periods. Furthermore, AI-driven tools like NeuralGCM currently lack integration of essential physical principles, limiting their capacity to address issues on land effectively.
However, AI’s potential in climate science extends beyond direct forecasting. Many models must rely on predefined scenarios of social and political behavior, which, while simplifying evaluations, can be inflexible and vague. AI can improve upon this approach by providing dynamic tools known as emulators that allow users to adapt models based on specific needs. These emulators are already in use among urban planners, insurers, and agricultural firms to make tailored predictions based on larger climate models. In 2023, researchers from the Commonwealth Scientific and Industrial Research Organisation (CSIRO) in Australia developed an emulator capable of recalibrating predictions based on emission levels a million times faster than its parent model. The growing precision and adaptability of these models are critical for making them accessible and beneficial for those confronting climate challenges on the ground.
The Intersection of AI and the Energy Sector
Simultaneously, AI is transforming another sector critical to climate change: the energy industry. At ADIPEC 2024 in Abu Dhabi, where 180,000 attendees from the global oil and gas sector gathered, the spotlight was on AI’s potential to increase efficiency while reducing greenhouse gas emissions. ADNOC CEO Sultan Al Jaber convened leaders from both tech and energy sectors to discuss AI’s future impact, which was widely anticipated to help streamline operations, cut emissions, and meet rising energy demands. However, many industry leaders are looking at more immediate benefits, particularly in the form of natural gas demand to power data centers, driven by the growing computational needs of the AI boom.
Data centers require massive amounts of energy, and Goldman Sachs predicts that the United States alone will need an additional 47 gigawatts of power capacity by 2030 to meet these demands, with 60% likely sourced from natural gas. The intersection of AI and natural gas presents a significant environmental challenge, as tech companies that are vocal about net-zero commitments may have to rely on gas in the short term, risking their pledges in favor of staying competitive in the AI-driven tech race. Recent reports reveal that upstream processes in the natural gas industry, including methane flaring and venting, contribute significantly to greenhouse gas emissions, contrary to industry claims that gas is cleaner than coal. Methane is notably potent, trapping significantly more heat in the atmosphere than carbon dioxide, further complicating the clean energy narrative of natural gas.
The environmental challenges associated with natural gas usage place tech companies in a difficult position: to meet their sustainability goals, many are investing in clean energy development. For example, Microsoft entered a $10 billion agreement with Brookfield, a global asset manager, to develop 10 gigawatts of renewable energy sources to power its data centers. However, achieving net-zero emissions while sustaining the pace of AI innovation remains an elusive goal. Even if tech companies secure sufficient clean energy for their own needs, they may still inadvertently prolong the use of gas for other industries by monopolizing the limited clean-energy capacity available, thereby extending the reliance on gas across other sectors.
AI in the Fight Against Climate Change
The AI boom presents both risks and opportunities in the battle against climate change. On one hand, AI-based climate models could lead to breakthroughs in prediction accuracy and resilience planning, while emulators offer localized solutions for cities and sectors. On the other hand, the energy needs for advancing AI could drive a counterproductive increase in natural gas consumption, entrenching fossil fuel use at a time when transitioning to renewables is critical. As world leaders debate these issues in Baku, and energy executives meet in Abu Dhabi, the challenges of balancing technological advancements with environmental sustainability remain at the forefront. AI may be a powerful tool in the climate response, but its environmental footprint and the limitations of current technologies remind us of the complex trade-offs involved in both combating and adapting to climate change.
Comments